Better models needed to predict risk of atrial fibrillation from medical records
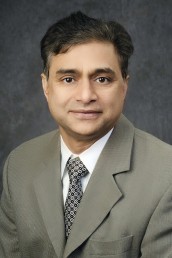
Dr. Dawood Darbar is chief of cardiology at UI Health and professor of medicine and pharmacology in the UIC College of Medicine
An ounce of prevention is worth a pound of cure. That’s why Dr. Dawood Darbar, chief of cardiology at the University of Illinois Hospital & Health Sciences System, worked with a team of physicians and researchers to answer one important question: Will a widely accepted atrial fibrillation risk prediction algorithm work when applied to the electronic medical record?
Early identification of patients at risk for atrial fibrillation is essential, Darbar said, to reduce the common and severe complications of stroke, heart failure and death from atrial fibrillation, the most common type of irregular heartbeat.
Risk prediction, he said, should leverage the technology routinely used in clinical practice.
“The electronic medical record is increasingly pervasive in clinical practice, and it is a powerful tool in patient care,” said Darbar, who is also professor of medicine and pharmacology in the UIC College of Medicine. “To be effective, risk prediction models need to work when applied to EMRs, where they are most likely to be used by physicians to identify subjects at risk for developing a disease.”
In a study published in the journal JAMA Cardiology, Darbar and colleagues found that risk prediction models for atrial fibrillation developed by investigators on the Cohorts for Heart and Aging Research in Genomic Epidemiology (CHARGE) trial, did not accurately predict incidence of the condition when it was applied to the EMRs of a large group of patients.
The study looked retrospectively at the medical records of patients who as of December 2005 did not have atrial fibrillation but returned for follow-up care at least three times within the next two years. Researchers used the EMR to follow their health for five years (through December 2010) to see how many developed atrial fibrillation.
They found that the CHARGE-AF risk model, when applied to the EMR, underpredicted the incidence of atrial fibrillation among low-risk subjects — and overpredicted the incidence among high-risk subjects.
“This study illustrates the challenges of applying a predictive model developed in prospective cohort studies to a real-world EMR setting,” Darbar said. “Ultimately, as health care advances, so will the role of the EMR. It follows that risk models should be derived from and validated in EMR cohorts, with an ultimate goal of developing individualized preventive strategies.”
The study suggests the model’s failure to accurately predict atrial fibrillation may be due to the different baseline characteristics of the prospective and EMR cohorts. Darbar and his co-authors also acknowledge some limitations of the study, including inconsistent EMR data-entry procedures, and an “indication bias,” wherein individuals who developed atrial fibrillation likely had more clinical encounters than those who did not.
The U.S. Centers for Disease Control and Prevention estimate that up to 6.1 million people in the United States have atrial fibrillation and that the condition contributes to approximately 130,000 deaths each year.
Co-authors on the paper include Dr. Matthew J. Kolek, Amy J. Graves, Meng Xu, Aihua Bian, Pedro Luis Teizeira, Dr. M. Benjamin Shoemaker, Dr. Babar Parvez, Dr. Joshua C. Denny, Frank E. Harrel and Dr. Dan Roden from the Vanderbilt University School of Medicine; Hua Xu of the University of Texas at Houston; Susan R. Heckbert of the University of Washington; Dr. Patrick T. Ellinor of Massachusetts General Hospital; Dr. Emelia J. Benjamin of Boston University; Dr. Alvaro Alonso of Emory University; Karel G.M. Moons of University Medical Center Utrecht in the Netherlands; and Ayumi K. Shintani of Osaka University in Japan.
This study was funded by Clinical and Translational Science Award UL1TR000445 from the National Center for Advancing Translational Sciences, the Cohorts for Heart and Aging Research in Genomic Epidemiology Challenge, and grants 1RC1HL101056, 2R01HL092577, 2R01HL092217, and N01HC25195 from the National Heart, Lung, and Blood Institute.