Researchers generate a carbon capture breakthrough using AI, physics and supercomputers
As the pace of climate change accelerates, the need for effective, scalable carbon-capture technologies is becoming increasingly urgent. But inventing new materials, like those needed to remove carbon emissions from the atmosphere, is often a slow, iterative process.
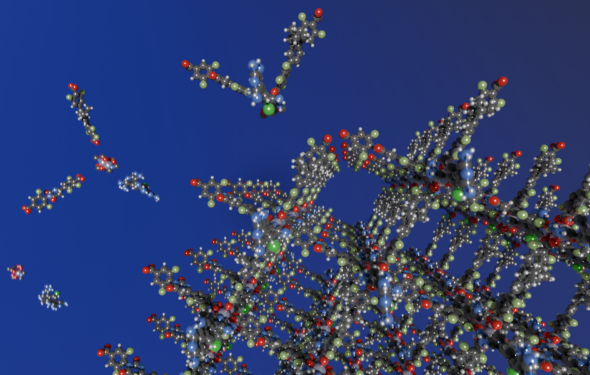
To increase the tempo of discovery, researchers at University of Illinois Chicago, Argonne National Laboratory and several other institutions are collaborating to harness the same artificial intelligence approaches that power recently hyped systems that create images from text prompts.
“The race for capturing carbon hinges on finding needles in a haystack, and trial and error is too slow. You have billions and billions of possibilities, and then you must narrow down to candidates that are good carbon absorbers,” said Santanu Chaudhuri, professor of civil, materials and environmental engineering at UIC and director of manufacturing science and engineering at Argonne. “With this project, we have taken the first significant step towards closing that gap by using generative AI.”
The open-source framework, described in a new Nature Communications Chemistry paper, co-authored by Chaudhuri and Xiaoli Yan of UIC, produced six promising candidates for carbon capture to explore further.
The research team’s AI model delves into the vast space of potential chemical arrangements that could be used to create materials called metal-organic frameworks and offers promising structures for further investigation. After the model suggested tens of thousands of potential candidates, the team tested the physical properties of the structures and their ability to capture carbon molecules in a series of computational models.
Metal-organic frameworks are modular materials made up of three kinds of building blocks: inorganic nodes such as zinc or copper; organic nodes; and molecular linkers made up of carbon, oxygen and other elements. The team’s AI model learned from a dataset of hypothetical metal-organic frameworks and suggested new molecular linkers predicted to have high capacity for capturing carbon from the air.
In all, the model assembled 120,000 possible structures. The team then ran through additional tests to remove those with improbable physical or chemical features or those that would be too difficult or expensive to make. The researchers also used computer models to predict each structure’s carbon capture abilities and subjected the best 364 candidates to in-depth, 3D molecular dynamics simulations of their structural qualities.
“We built a series of funnels, and we gradually decrease the involvement of AI and increase the physics and chemistry at each step,” said Yan, a PhD student in materials engineering at UIC and Argonne. “By doing so, we can evaluate these organic molecules in terms of chemical validity, synthesizability and stability.”
Finally, the authors arrived at the six highest-performing structures featured in the paper. These structures can be synthesized in laboratories and subjected to real-world experiments, while the data from the computational tests can be fed back into the AI model to produce another generation of even higher-quality candidates.
All told, the entire framework, from AI model to 3D simulations, can be completed in 12 hours using modern supercomputing resources, the authors said. The framework and its components will also be open-source, available for the scientific community to replicate or adapt for other metal-organic framework applications.
“We want to release the power and the imagination of a large community of different researchers because carbon capture is an urgent need,” Chaudhuri said. “If you keep it to yourself, then you are reducing the chance of innovation.”
The research was done in collaboration with Eliu Huerta, Lead for Translational AI at Argonne, part of a growing research partnership between UIC and Argonne. Computational work for the paper was performed at the Argonne Leadership Computing Facility and the National Center for Supercomputing Applications at the at the University of Illinois Urbana-Champaign. The work was supported by grants from the Department of Energy and the National Science Foundation. More information about the project is available online.